Research Q&A: Creating Adjusted Mobility Scores and Benchmarks in Lower Limb Prosthetic Care
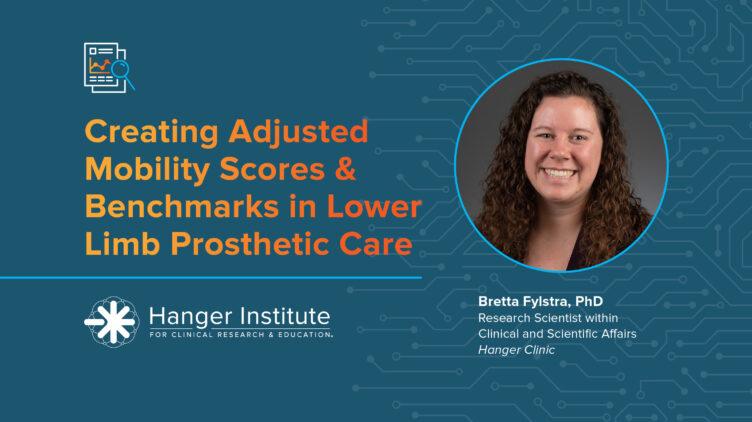
We sat down with Bretta Fylstra, PhD, Research Scientist with Clinical and Scientific Affairs at Hanger Clinic, to better understand the real-world clinical implications of the CASTLE study, which establishes mobility benchmarks for people with lower limb amputation based on age and etiology.
CASTLE 1: Determination of Normative Mobility Scores After Lower Limb Amputation for Each Year of Adulthood
Results offer tools for determining average mobility based on age and amputation level for the most common lower limb amputation etiologies of trauma and diabetes / dysvascular (DV).
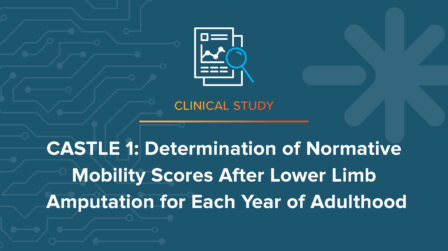
Why did the Hanger Institute decide to embark on the CASTLE study?
CASTLE expands on the MAAT 7 clinical study that looked at the four most common etiologies and changes in mobility across seven age groups. In MAAT 7, age was grouped into ten-year bands. With CASTLE, we get a more granular perspective, looking at each age group individually and diving deeper into how mobility changes based on etiology and with each year of age.
What is the goal behind the CASTLE study?
We set out to better understand an amputee’s mobility as they age, with the expectation that different variables will have a varying impact on mobility. We researched amputation level, cause of amputation, age, gender, and device type.
Expanding our understanding of these specific patient presentations across every year of adulthood can assist with monitoring a patient’s trajectory and benchmarking performance for the majority of patients requiring lower limb prosthetic care. As clinicians, this is important to understanding patient progress, evaluating outcomes, and moving towards value-based care.
Why is the CASTLE study important to clinicians and the prosthetic field?
CASTLE helps to set benchmarks across each year of adulthood while also supporting adjusted mobility scores. With an adjusted mobility score, prosthetists are able to generate predicted mobility scores to qualify good outcomes in lower limb prosthetic care. Typically, we would consider 50 to be average. But in the lower limb prosthetic population, when looking at a specific attribute, average may actually be 41. This is an adjusted mobility score. If a patient then scores 60, we would know they are performing significantly above average compared to their peers versus slightly above average. We can then assign an adjusted mobility score to capture just how well this patient is doing compared to their peers.
All of this equates to better goal setting for patients with a better understanding of where we can expect them to be.
What does this all mean for lower limb prosthetic patients?
For lower limb prosthetic patients, it allows them to set expectations and get a better sense of how they are doing relative to people similar to themselves. They are able to specifically compare how they are performing to their peers in their age group and with similar characteristics, and not compare themselves to the general population.
How can this study potentially have an impact in the payor community?
The United States healthcare system is transitioning from traditional fee-for-service models to value-based care, creating an increased need to demonstrate quality care through clinical outcomes. As we shift towards this idea of reimbursement based on value, we need benchmarks, so we have a good understanding of where we expect patients to be. The CASTLE study helps continue to create these benchmarks and supports adjusted mobility scores.
Anything additional from your work on CASTLE you would like to share?
In our secondary analysis, we also took the opportunity to look at device type and compared above-knee amputees with a microprocessor knee (MPK) versus a non-microprocessor knee (nMPK). This is an exciting opportunity to explore the impact that technology may have on patients.
For both trauma and diabetes/dysvascular (DV) etiologies, patients with an MPK have higher PLUS-M scores compared to those with a nMPK. Further, for patients with DV etiology, the MPK may slow the decrease in mobility relative to age.
Anything you enjoyed during your work on this study from a personal perspective?
My background is engineering. With past studies and observations, the data sets we analyzed were much smaller. This study had a data set of 29,522 individuals. It was so interesting to explore the data from a population level and see these larger trends.
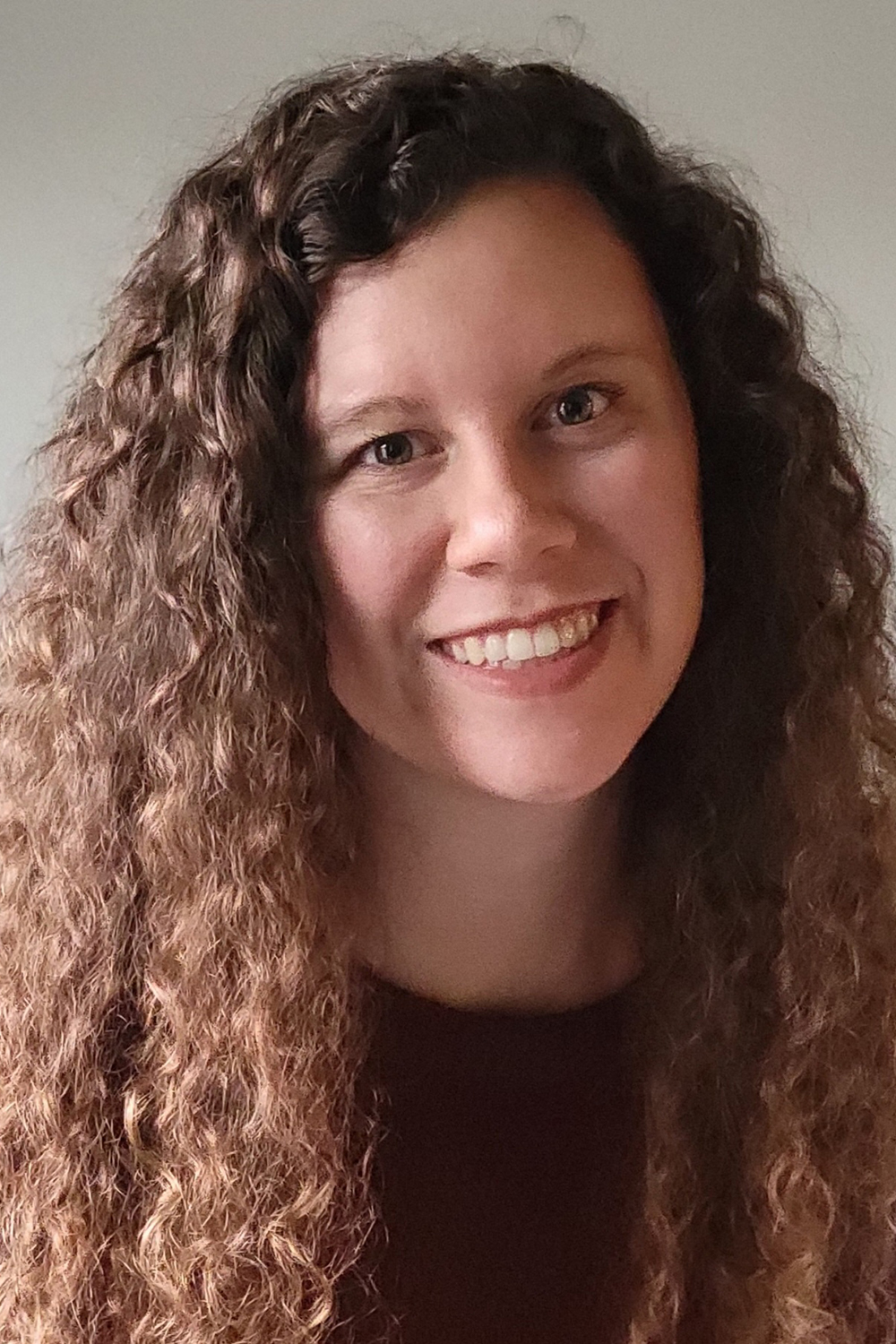
More Published Research
Review additional studies conducted by the Hanger Institute for Clinical Research and Education, in collaboration with leading researchers, clinical, and academic institutions.
Latest Updates
Subscribe to stay up-to-date on our latest posts.